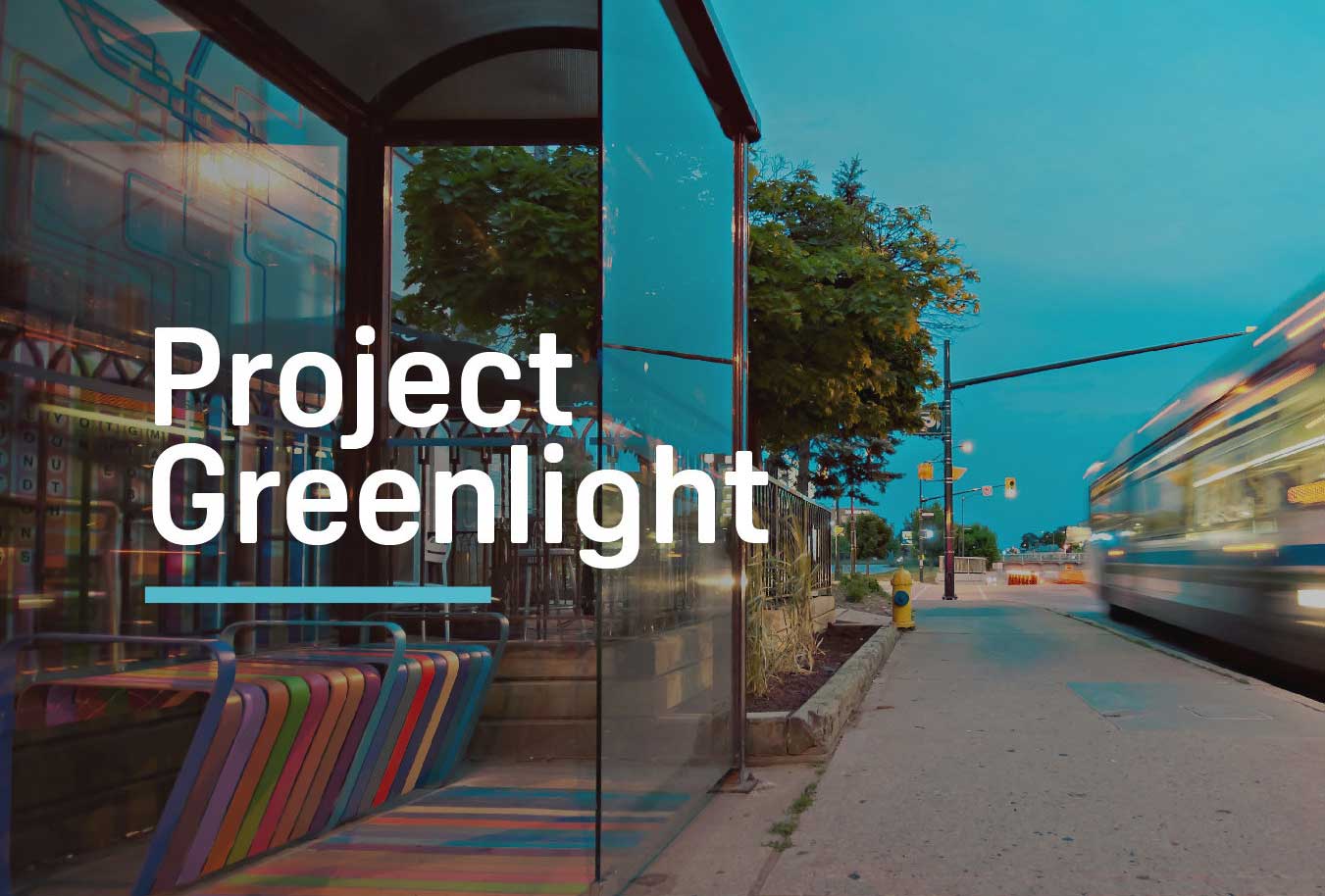
Scope Snapshot
Goal
To ingest FortisBC’s GIS and time-series data to create an Energy Data Model that the PwC team could easily access to enable to development of algorithms to provide new AI-driven phase and meter-to-transformer connectivity correction algorithms on a specific set of FortisBC feeders.
Data
One substation with six feeders for a total of 10,593 meters.
Awesense Tools Used
The Energy Transition Platform and its subcomponents, including the AI Data Engine, Awesense Energy Data Model APIs & the Awesense True Grid Intelligence (TGI) for asset and grid edge situational awareness.
- Low-quality data for meter-to-transformer association and meter-to-phase assignation
- Inability to serve and provide cleansed, structured data from a single platform in a collated format for use case development
- While seeing the potential of the data, they needed help with developing solutions and use cases through a unified platform using FortisBC GIS and time-series data due to extensive requirements for bringing disparate data sources together.
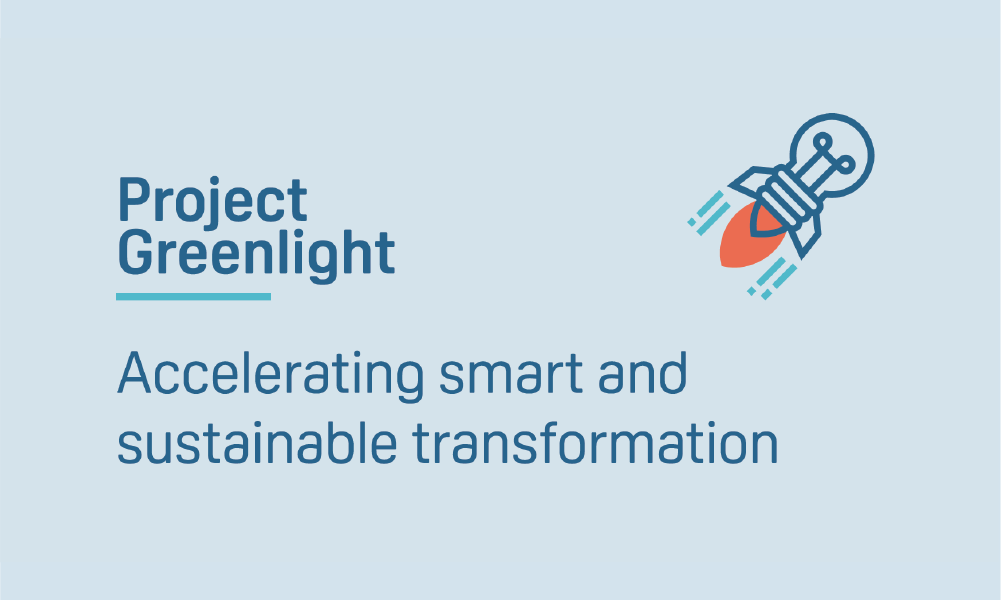